Your source for everything mobile UA, from the basics to contentious standards, the glossary can help and inform both aspiring growth managers and experienced mobile app developers
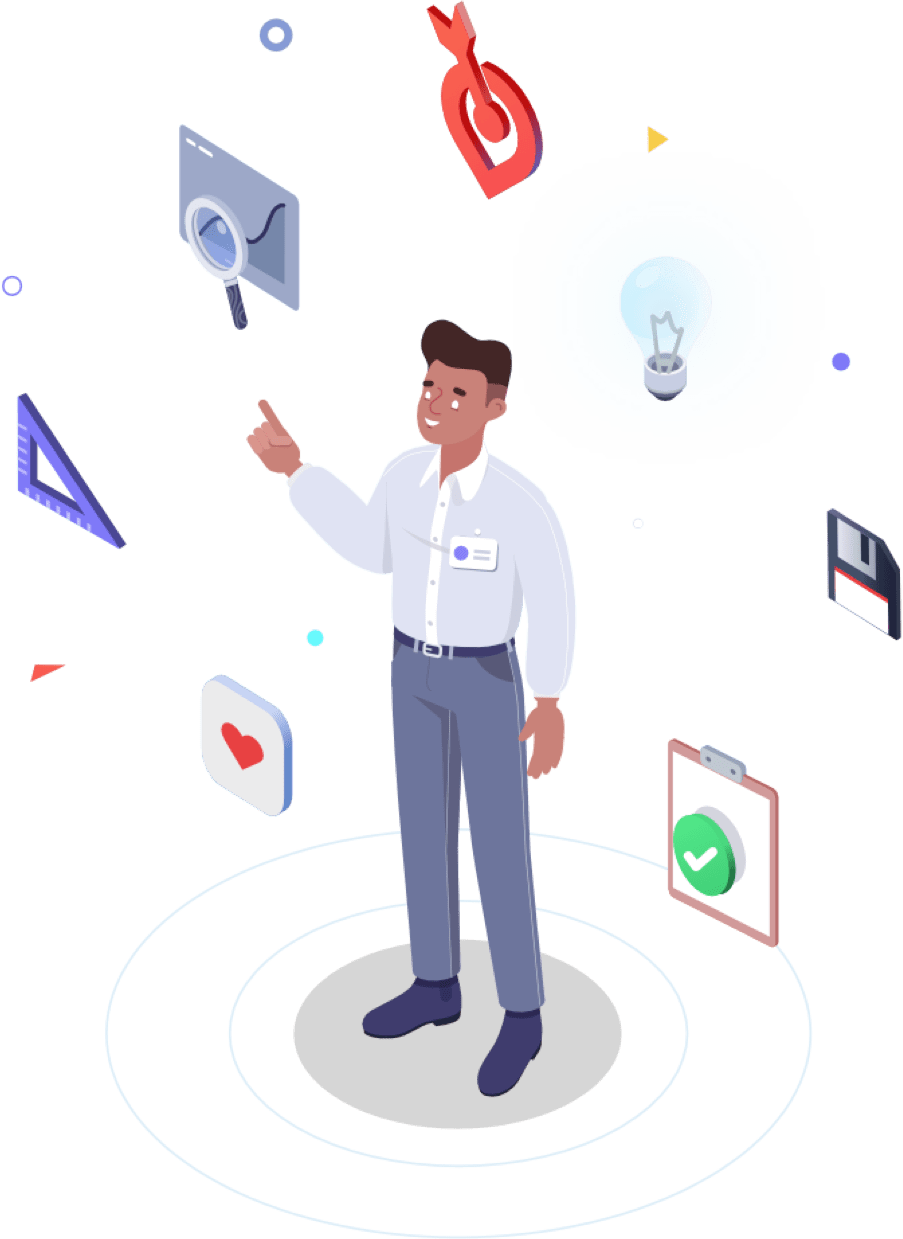
Real-time bidding
Data Sharing in Mobile Marketing -> Page 1 of
Data Sharing in Mobile Marketing
What is Data Sharing in Mobile User Acquisition?
Finding Your Target Audience
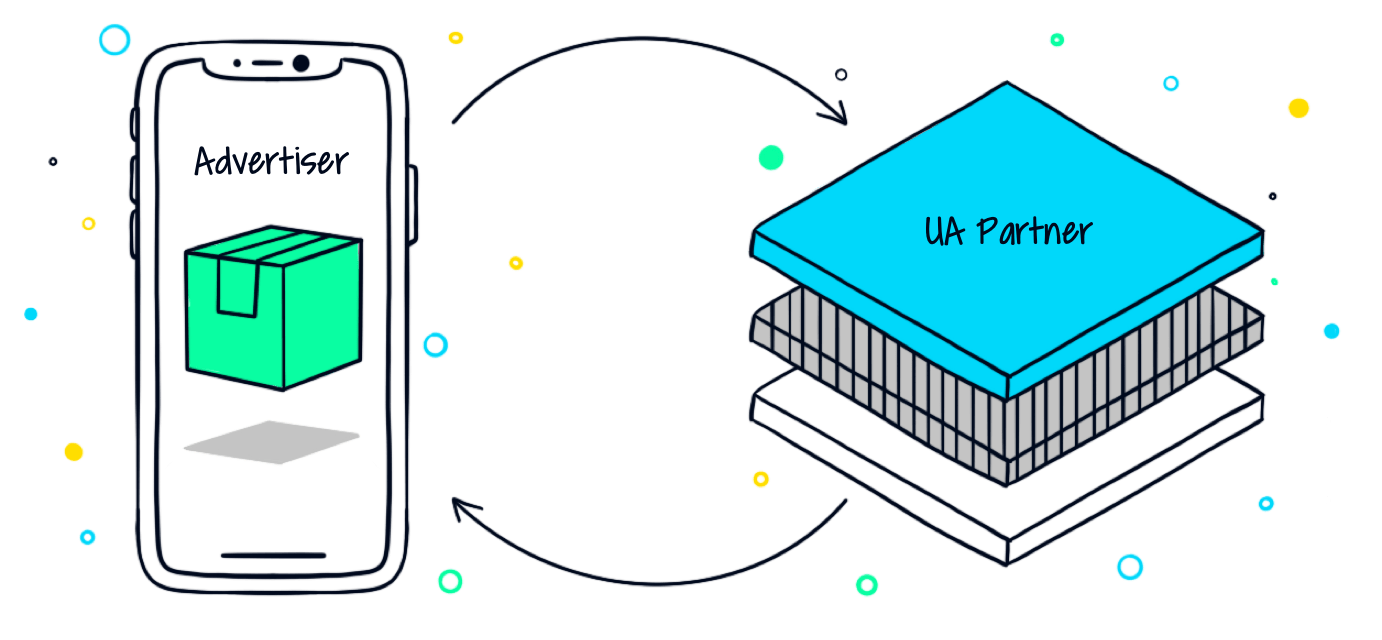
Data sharing in mobile UA means providing your UA partner access to essential UA-related data, in the form of an audience list, comprised of existing users. Sharing this data fulfills two central goals: it helps your UA partner target lookalikes, which, in turn, helps improve your campaign results, and it serves as a suppression list (formerly known as a blacklist) to prevent targeting existing users.
By using this data your campaigns are focused on acquiring new and relevant users, instead of wasting your budget on a long exploration phase and targeting existing and active users, or ones that already used your app and didn’t retent.
Sharing an audience list with your UA partner is relevant to partners who run data-driven UA campaigns. If the campaigns run by your partner are not, at the very least, programmatic, then sharing the data will only be used as a suppression list.
A Holistic Approach to Mobile UA
Using an Audience List to Target Lookalikes
While it may sound abrasive, data sharing has a huge impact on mobile marketing campaigns when they are run through a DSP partner that employs machine-learning. Sharing an audience list means that from the early stages of the campaign, your DSP partner has the necessary information required to target lookalikes (given that the partner has the technical capability to do so).
Using existing audience data can help your partner create a profile of a potential new user. Depending on the UA partner’s targeting abilities and its existing data, this data can help recognize behavioral patterns from which it can build an initial targeting model.
Some of the patterns that can be detected and targeted with are types of devices, OS versions, session depths, the time of day, and so on. Recognizing common behaviors between existing users allows for the creation of a targeting model (programmatic or fully based on machine learning) which, in turn, learns based on its performance.
For example, let’s say the app at hand is a hyper-casual gaming app. You provide your UA partner with data about your existing users (i.e an audience list). Your partner uses this data to detect patterns and sees a specific time-of-day activity, a repeating average session length, and that these engaged users all are highly active in the hyper-casual genre. They might also recognize a more engaged gender or age group. These patterns can be picked up by the machine learning model and guide bidding decisions, to ensure only users with a higher probability to install and engage with the app are bid on.
Optimizing Your UA Campaign - From Targeting Lookalikes to Reaching KPIs
Using the audience list, your partner can start the campaign by targeting only relevant users. Now, the algorithm can learn and improve based on the performance of the initial lookalike targeting campaigns, according to the set KPIs, and optimize towards users with similar behavioral patterns and target them exclusively. This process can be thought of as sort of a refinement of the model, in which the model is already set on targeting the high-intent users, tracking their performance, and optimizing towards the set KPIs.
To put it simply, thanks to the data shared, the campaign starts by targeting lookalikes, and then, as it learns and tracks data trends and the goal KPIs, a targeting model is established and its targeting keeps improving over time, as the model learns from its past mistakes (hence the learning in Machine Learning). If earlier we’ve mentioned the type of device, and interest in the genre, now it may find even more distinctive behavioral patterns common to most ideal users (those users may be high retention users, depositors, or something else, depending on the set KPIs).
Read more on data activation in our Data Activation in Mobile UA entry.
This is a win-win-win situation, for the advertiser, that sees results faster, the DSP, that can model and scale faster, and the users, that are shown ads relevant to their interests. Having said that, sharing first-party data is not a matter to be taken lightly, and should only be shared with a trusted partner.
The Different Types of Audience Lists
There are two important distinctions when it comes to sharing audience lists – the type of list (dynamic or static) and content segmentation.
- Static lists
As the name suggests, these lists are a single delivery (i.e, they’re given one time). They consist of an existing list of all users up to a certain point in time. If you’re running other UA campaigns at the same time, either on social networks or with other UA partners, the static nature of the list may cause problems, depending on the existing pool of users and the scale of the campaign. A static list may turn irrelevant if the app is new and the users’ pool is relatively small, or in cases in which multiple campaigns are running parallelly on different platforms. In general, a static list is not an ideal choice, but it’s still better than not sharing a list at all.
- Dynamic lists
Dynamic lists update automatically, as new users install the app, regardless of where the install originated. This is useful (and highly recommended) when running multiple UA campaigns on different platforms and helps avoid targeting newly acquired users.The different attribution platforms can help create and maintain these lists (and even segment these lists) by offering their users a very simple way to share these ongoing, dynamic audience lists with their various UA partners. This feature not only benefits the performance of specific immediate UA campaigns by ensuring newly acquired users are not targeted, but it also allows your partners to gain a truly holistic overview of your user base, which in turn can significantly impact performance for the long run.
- Custom/Segmented Audience list
These lists, which can be either static or dynamic, are segmented by user behavior, expressed in in-app events – users that reached D30 retention, inactive users, depositors, inactive depositors, and so on. These segmentations can be used by a UA partner to recognize data patterns faster and target accordingly. If the campaign KPI is D30 retention, for example, your partner can look at a D30 retention segment and recognize data patterns specific to these users (such as type of device, OS version, depth of session, app genres, active hours, etc’) and target lookalikes. The segmentation helps your partner find patterns that are distinctive to the segment and improve their targeting. If the campaign is ML-based, and the list is dynamic and segmented, then as data patterns shift and change so would the model (if something in the patterns of D30 retention users changed so would, consequently, the targeting). If these segmentations don’t exist, but your partner is still running an ML-based campaign, these data patterns will eventually be surmised, but it may take significantly longer to collect enough samples.
Is an Audience List the Same Thing as a Suppression List?
A suppression list is a term mostly used in the context of email marketing, where it’s commonly used in order to avoid targeting existing users. In the context of data-driven mobile UA, they’re used similarly and help focus the spending budget on acquiring new users. We’re avoiding the term suppression list since data sharing (or audience lists) has a bigger contribution and functionally in mobile UA campaigns than suppression lists.
Customizing Ad Serving According to the Audience Segment
The Effect of Creative Selection
Once the data is shared and the audience is segmented, we can delve into creative selection. Customizing the creatives and adapting them to relevant audience segments can significantly improve the campaign’s performance.
For example, using a playable ad or an introductory video ad can work well when introducing the app to a completely new user. Whereas, when retargeting users, there’s no point in introducing them to the app, but rather expose them to newly available content, or content they haven’t interacted with yet.
When it comes to retargeting campaigns, there are almost endless options for customization. It can be customized down to the stage users reached in the app or the specific product they were about to purchase.
For example, if a user reads the product description but didn’t add it to the cart, vs. a user that added to the cart but didn’t complete the purchase. The first user can get an ad with a notification that the product is still available, whereas, since the second user was further in the funnel, this ad would be an incentivized ad offering a discount for completing the purchase.
The ability to customize at such a granular level can lead to better KPIs and more engaged users. The options are limitless, and as such, should be used in moderation (don’t scare off your users by offering them 5 ads a day, each one detailing exactly where they abandoned the app).